Computers & Graphics, Vol. 53(A), December 2015
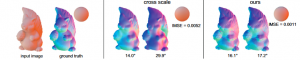
“A discriminative approach to perspective shape from shading in uncalibrated illumination”- Stephan R. Richter, Stefan Roth TU Darmstadt – Estimating surface normals from a single image alone is a challenging problem. Previous work made various simplifications and focused on special cases, such as having directional lighting, known reflectance maps,etc. This is problematic, however, as shape from shading becomes impractical outside the lab. We argue that addressing more realistic settings requires multiple shading cues to be combined as well as generalized to natural illumination. However, this requires coping with an increased complexity of the approach and more parameters to be adjusted. Starting from a novel large-scale dataset for training and analysis, we pursue a discriminative learning approach to shape from shading. Regression forests enable efficient pixel-independent prediction and fast learning. The regression trees are adapted to predicting surface normals by using von Mises–Fisher distributions in the leaves. Spatial regularity of the normals is achieved through a combination of spatial features, including texton as well as novel silhouette features. The proposed silhouette features leverage the occluding contours of the surface and yield scale-invariant context. Their benefits include computational efficiency and good generalization to unseen data. Importantly, they allow estimating the reflectance map robustly, thus addressing the uncalibrated setting. Our method can also be extended to handle perspective projection. Experiments show that our discriminative approach outperforms the state of the art on various synthetic and real-world datasets. PDF